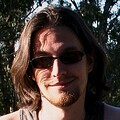
tvanderpol
Genetic Algorithms in Elixir: First stab at Ch1 algorithm converges just fine? (p28)
I’ve got something of the opposite of the usual problem - my code doesn’t fail in the way the text suggests it should (when it suggests premature convergence is the cause).
I’ve ran the code a fair bit and did some debug print injecting and such but I can’t really see anything out of the ordinary - it converges on the correct answer almost instantly if I run it without any additional debug and it takes a reasonable amount of generations from what I can tell by poking at it.
Now I am clear to continue the chapter as-is, and I understand the argument being made, but I don’t understand how the algorithm is meant to fail (I do in the abstract but I mean the code as written) and that’s bugging me.
Marked As Solved
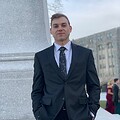
seanmor5
There was a mistake in my version of the code that forced early convergence with smaller populations that isn’t present in the book’s transcription of the code.
To better demonstrate premature convergence: set chromosome size to 1000 and population size to 100. You’ll notice your version without mutation converges much slower than with mutation. You can continue to decrease the population size further and further and you’ll reach a point where progress completely stops.
Sorry about the confusion!
Also Liked
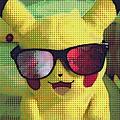
christhekeele
This was on quite a new Macbook, which may be influencing results from what’s expected.
MacBook Pro (16-inch, 2019)
2.4 GHz 8-Core Intel Core i9
32 GB 2667 MHz DDR4
Erlang/OTP 22 [erts-10.7.2] [64-bit] [smp:16:16]
Elixir 1.10.3
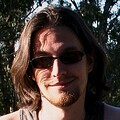
tvanderpol
Perfect, thank you for responding so fast! That helps my understanding of exactly how it fails a lot.
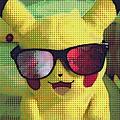
christhekeele
I noticed this in the B1 edition as well!
Re:
$ elixir one_max.exs Current Best: 32
But wait, what’s going on here? Why is the algorithm stopping on a best fitness below 42? No matter how many times you run it, the algorithm will almost always certainly stop improving below 42. The problem is premature convergence.
At this point, the chapter’s example code looks like:
Code to Date
population = for _ <- 1..10, do: for _ <- 1..42, do: Enum.random(0..1)
evaluate = fn population ->
Enum.sort_by(population, &Enum.sum(&1), &>=/2)
end
selection = fn population ->
population
|> Enum.chunk_every(2)
|> Enum.map(&List.to_tuple(&1))
end
crossover = fn population ->
Enum.reduce(population, [], fn {p1, p2}, acc ->
cx_point = :rand.uniform(42)
{{h1, t1}, {h2, t2}} = {Enum.split(p1, cx_point), Enum.split(p2, cx_point)}
[h1 ++ t2 | [h2 ++ t1 | acc]]
end)
end
algorithm = fn population, algorithm ->
best = Enum.max_by(population, &Enum.sum(&1))
IO.write("\rCurrent Best: " <> Integer.to_string(Enum.sum(best)))
if Enum.sum(best) == 42 do
best
else
population
|> evaluate.()
|> selection.()
|> crossover.()
|> algorithm.(algorithm)
end
end
solution = algorithm.(population, algorithm)
IO.write("\n Answer is \n")
IO.inspect solution
I parameterized it thusly:
Tunable version
-population = for _ <- 1..10, do: for _ <- 1..42, do: Enum.random(0..1)
+problem_size = 42
+population_size = 100
+
+population = for _ <- 1..population_size, do: for _ <- 1..problem_size, do: Enum.random(0..1)
evaluate = fn population ->
Enum.sort_by(population, &Enum.sum(&1), &>=/2)
end
selection = fn population ->
population
|> Enum.chunk_every(2)
|> Enum.map(&List.to_tuple(&1))
end
crossover = fn population ->
Enum.reduce(population, [], fn {p1, p2}, acc ->
- cx_point = :rand.uniform(42)
+ cx_point = :rand.uniform(problem_size)
{{h1, t1}, {h2, t2}} = {Enum.split(p1, cx_point), Enum.split(p2, cx_point)}
[h1 ++ t2 | [h2 ++ t1 | acc]]
end)
end
algorithm = fn population, algorithm ->
best = Enum.max_by(population, &Enum.sum(&1))
IO.write("\rCurrent Best: " <> Integer.to_string(Enum.sum(best)))
- if Enum.sum(best) == 42 do
+ if Enum.sum(best) == problem_size do
best
else
population
|> evaluate.()
|> selection.()
|> crossover.()
|> algorithm.(algorithm)
end
end
solution = algorithm.(population, algorithm)
IO.write("\n Answer is \n")
IO.inspect solution
In my experimentation, with problem_size = 42
, not only did population_size = 100
always converge on the best answer, but even as low as population_size = 8
consistently converged. I started seeing the need for mutation around population_size = 6
, which normally gets stuck around 35
.
Alternatively, increasing the size of the problem to problem_size = 420
usually converged correctly, but with enough time to watch things work. problem_size = 4200
consistently gets stuck around 2400
, as the narrative of the chapter wants it to.
Popular Pragmatic Bookshelf topics
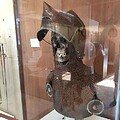
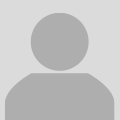
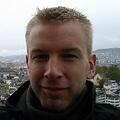
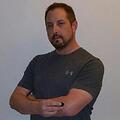
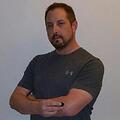
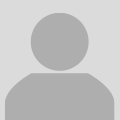
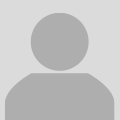
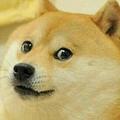
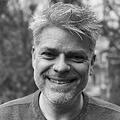
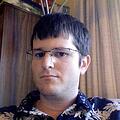
Other popular topics
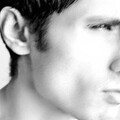
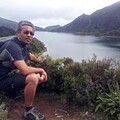
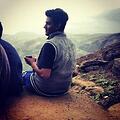
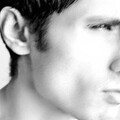
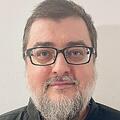
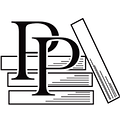
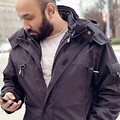
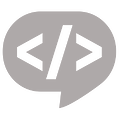
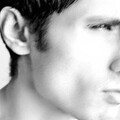
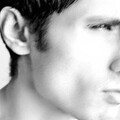
Categories:
Sub Categories:
Popular Portals
- /elixir
- /rust
- /wasm
- /ruby
- /erlang
- /phoenix
- /keyboards
- /rails
- /js
- /python
- /security
- /go
- /swift
- /vim
- /clojure
- /java
- /haskell
- /emacs
- /svelte
- /onivim
- /typescript
- /crystal
- /c-plus-plus
- /tailwind
- /kotlin
- /gleam
- /react
- /flutter
- /elm
- /ocaml
- /ash
- /vscode
- /opensuse
- /centos
- /php
- /deepseek
- /scala
- /html
- /zig
- /debian
- /nixos
- /lisp
- /agda
- /sublime-text
- /react-native
- /textmate
- /kubuntu
- /arch-linux
- /revery
- /ubuntu
- /manjaro
- /spring
- /django
- /diversity
- /nodejs
- /lua
- /c
- /slackware
- /julia
- /neovim