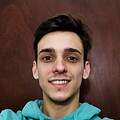
augusto1024
Machine Learning in Elixir: Chapter 7 - Low accuracy and weight matrix full of NaNs in MLP example
I’m going through the MLP Livebook for identifying cats and dogs, and after training the MLP model and testing it, I get an accuracy of 4.8 (way lower than the example in the book) and the weights matrix int he trained model state is full of NaNs. The code is exactly the same as in the book. What am I doing wrong?
Here’s the output for the trained model state:
%{
"dense_0" => %{
"bias" => #Nx.Tensor<
f32[256]
EXLA.Backend<host:0, 0.3457734646.1776680978.228705>
[-0.006004911381751299, NaN, NaN, -0.006001265719532967, -0.006005018018186092, NaN, NaN, NaN, -0.006005273200571537, -0.005989077966660261, NaN, NaN, NaN, -0.006004870403558016, NaN, NaN, -0.006005257833749056, -0.006004877854138613, -0.006005317438393831, NaN, -0.005980218760669231, -0.005973377730697393, -0.00600520521402359, NaN, NaN, NaN, -0.006004676688462496, NaN, NaN, NaN, NaN, -0.006004626862704754, NaN, -0.006004307884722948, NaN, -0.006003706716001034, NaN, -0.006005176343023777, NaN, NaN, -0.00600530905649066, NaN, -0.006003919057548046, -0.005942464806139469, NaN, -0.006004999857395887, NaN, NaN, ...]
>,
"kernel" => #Nx.Tensor<
f32[27648][256]
EXLA.Backend<host:0, 0.3457734646.1776680978.228706>
[
[-0.009822199121117592, NaN, NaN, -0.019302891567349434, 0.0013210634933784604, NaN, NaN, NaN, -0.0035181990824639797, -0.003965682815760374, NaN, NaN, NaN, -0.012110317125916481, NaN, NaN, -0.010716570541262627, 0.006445782259106636, -0.005844426807016134, NaN, -0.008739138022065163, -0.009861554950475693, -0.01141569297760725, NaN, NaN, NaN, -0.007794689387083054, NaN, NaN, NaN, NaN, 0.007325031328946352, NaN, -0.008747091516852379, NaN, -0.015862425789237022, NaN, -0.0023863192182034254, NaN, NaN, -0.008942843414843082, NaN, -0.01665472239255905, -0.01721101626753807, NaN, -0.005523331463336945, NaN, ...],
...
]
>
},
"dense_1" => %{
"bias" => #Nx.Tensor<
f32[128]
EXLA.Backend<host:0, 0.3457734646.1776680978.228707>
[-0.006005339790135622, -0.006005363073199987, NaN, 0.0, -0.006005348637700081, -0.006000204011797905, NaN, -0.0059988489374518394, -0.00600522430613637, NaN, 0.0, 0.006004837807267904, NaN, NaN, 0.0059986296109855175, -0.006005391012877226, -0.006004904862493277, NaN, 0.0060051423497498035, NaN, 0.006003301590681076, NaN, NaN, NaN, -0.0060053858906030655, -0.006005320698022842, 0.0, 0.00600471580401063, 0.0, NaN, NaN, -0.006005088798701763, -0.0060053677298128605, NaN, NaN, -0.006004550959914923, NaN, -0.006004488095641136, -0.006004879716783762, NaN, NaN, NaN, NaN, NaN, 0.0, NaN, 0.006000214722007513, ...]
>,
"kernel" => #Nx.Tensor<
f32[256][128]
EXLA.Backend<host:0, 0.3457734646.1776680978.228708>
[
[0.1141437217593193, 0.02805522084236145, NaN, 0.09622809290885925, 0.05185674503445625, 0.017901137471199036, NaN, 0.046677932143211365, -0.12201476842164993, NaN, -0.09235477447509766, -0.006104507949203253, NaN, NaN, 0.08608447760343552, 0.012301136739552021, -0.05758747458457947, NaN, -0.08425487577915192, NaN, -0.07365603744983673, NaN, NaN, NaN, 0.07276518642902374, 0.00285704736597836, -0.12260323762893677, 0.11970219016075134, -0.08480334281921387, NaN, NaN, -0.039198994636535645, -0.03682233393192291, NaN, NaN, -0.08676794916391373, NaN, 0.03924785554409027, 0.07963936030864716, NaN, NaN, NaN, NaN, NaN, 0.027959883213043213, NaN, ...],
...
]
>
},
"dense_2" => %{
"bias" => #Nx.Tensor<
f32[1]
EXLA.Backend<host:0, 0.3457734646.1776680978.228709>
[NaN]
>,
"kernel" => #Nx.Tensor<
f32[128][1]
EXLA.Backend<host:0, 0.3457734646.1776680978.228710>
[
[NaN],
[NaN],
[NaN],
[NaN],
[NaN],
[NaN],
[NaN],
[NaN],
[NaN],
[NaN],
[NaN],
[NaN],
[NaN],
[NaN],
[NaN],
[NaN],
[NaN],
[NaN],
[NaN],
[NaN],
[NaN],
[NaN],
[NaN],
[NaN],
[NaN],
[NaN],
[NaN],
[NaN],
[NaN],
[NaN],
[NaN],
[NaN],
[NaN],
[NaN],
[NaN],
[NaN],
[NaN],
[NaN],
[NaN],
[NaN],
[NaN],
[NaN],
[NaN],
[NaN],
[NaN],
...
]
>
}
}
Most Liked
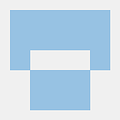
chico1992
HI, I ran into the same issues but was able to make it work by pinning the versions of axon, nx and elxa to the latest 0.5.x version and make the examples work the same way as in the book
{:axon, "== 0.5.1"},
{:nx, "== 0.5.3"},
{:exla, "== 0.5.3"},
hope this helps if someone else comes across this issue
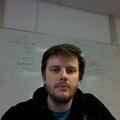
Christophe
Hello @seanmor5
I have the same problem, from chapter 7 when I try the cnn_trained_model_state the results are not the same as in the book :
09:03:50.990 [debug] Forwarding options: [compiler: EXLA] to JIT compiler
Epoch: 0, Batch: 150, accuracy: 0.5013453 loss: 7.5956130
Epoch: 1, Batch: 163, accuracy: 0.5018579 loss: 7.6527510
Epoch: 2, Batch: 176, accuracy: 0.5010152 loss: 7.6714020
Epoch: 3, Batch: 139, accuracy: 0.5034598 loss: 7.6697083
Epoch: 4, Batch: 152, accuracy: 0.5019404 loss: 7.6802869
And I have NaN in the model
[NaN],
[NaN],
[NaN],
[NaN],
[NaN],
[NaN],
[NaN],
[NaN],
...
]
>
}
}
```
Popular Pragmatic topics
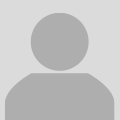
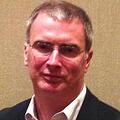
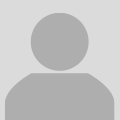
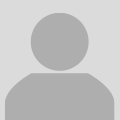
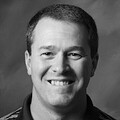
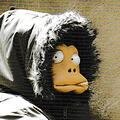
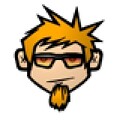
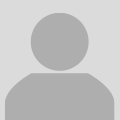
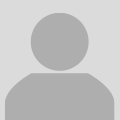
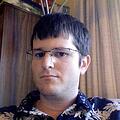
Other popular topics
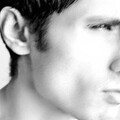
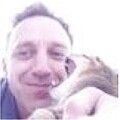
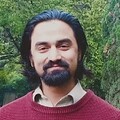
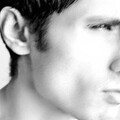
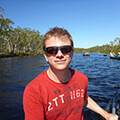
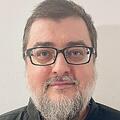
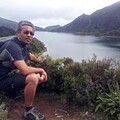
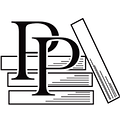
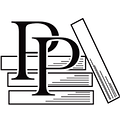
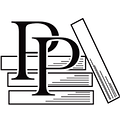
Latest in PragProg
Latest (all)
Categories:
Popular Portals
- /elixir
- /rust
- /wasm
- /ruby
- /erlang
- /phoenix
- /keyboards
- /js
- /rails
- /python
- /security
- /go
- /swift
- /vim
- /clojure
- /java
- /haskell
- /emacs
- /svelte
- /onivim
- /typescript
- /crystal
- /c-plus-plus
- /tailwind
- /kotlin
- /gleam
- /react
- /flutter
- /elm
- /ocaml
- /vscode
- /opensuse
- /ash
- /centos
- /php
- /deepseek
- /zig
- /scala
- /html
- /debian
- /nixos
- /lisp
- /agda
- /textmate
- /react-native
- /sublime-text
- /kubuntu
- /arch-linux
- /ubuntu
- /revery
- /manjaro
- /django
- /spring
- /diversity
- /nodejs
- /lua
- /julia
- /c
- /slackware
- /neovim