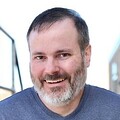
CoderDennis
Machine Learning in Elixir: chapter 7 CNN model accuracy no better than MLP (page 160)
When training the cnn_model, I get the following output:
Epoch: 0, Batch: 150, accuracy: 0.4985513 loss: 7.6424022
Epoch: 1, Batch: 163, accuracy: 0.4992854 loss: 7.6783161
Epoch: 2, Batch: 176, accuracy: 0.5000441 loss: 7.6865749
Epoch: 3, Batch: 139, accuracy: 0.4983259 loss: 7.6991839
Epoch: 4, Batch: 152, accuracy: 0.4988766 loss: 7.6995916
%{
"conv_0" => %{
"bias" => #Nx.Tensor<
f32[32]
EXLA.Backend<host:0, 0.1357844422.1979580433.82179>
[NaN, NaN, NaN, NaN, NaN, NaN, NaN, NaN, NaN, NaN, NaN, NaN, NaN, NaN, NaN, NaN, NaN, NaN, NaN, NaN, NaN, NaN, NaN, NaN, NaN, NaN, NaN, NaN, NaN, NaN, NaN, NaN]
>,
"kernel" => #Nx.Tensor<
f32[3][3][3][32]
EXLA.Backend<host:0, 0.1357844422.1979580433.82180>
[
[
[
[NaN, NaN, NaN, NaN, NaN, NaN, NaN, NaN, NaN, NaN, NaN, NaN, NaN, NaN, NaN, NaN, NaN, NaN, NaN, NaN, NaN, NaN, NaN, NaN, NaN, NaN, NaN, NaN, NaN, NaN, NaN, NaN],
[NaN, NaN, NaN, NaN, NaN, NaN, NaN, NaN, NaN, NaN, NaN, NaN, NaN, NaN, NaN, ...],
...
],
...
],
...
]
>
},
"conv_1" => %{
"bias" => #Nx.Tensor<
f32[64]
EXLA.Backend<host:0, 0.1357844422.1979580433.82181>
[NaN, NaN, NaN, NaN, NaN, NaN, NaN, NaN, NaN, NaN, NaN, NaN, NaN, NaN, NaN, NaN, NaN, NaN, NaN, NaN, NaN, NaN, NaN, NaN, NaN, NaN, NaN, NaN, NaN, -0.0071477023884654045, NaN, NaN, NaN, NaN, 0.0, NaN, NaN, NaN, NaN, NaN, NaN, NaN, NaN, NaN, NaN, NaN, NaN, ...]
>,
"kernel" => #Nx.Tensor<
f32[3][3][32][64]
EXLA.Backend<host:0, 0.1357844422.1979580433.82182>
[
[
[
[NaN, NaN, NaN, NaN, NaN, NaN, NaN, NaN, NaN, NaN, NaN, NaN, NaN, NaN, NaN, NaN, NaN, NaN, NaN, NaN, NaN, NaN, NaN, NaN, NaN, NaN, NaN, NaN, NaN, NaN, NaN, NaN, NaN, NaN, NaN, NaN, NaN, NaN, NaN, NaN, NaN, NaN, NaN, NaN, NaN, NaN, ...],
...
],
...
],
...
]
>
},
"conv_2" => %{
"bias" => #Nx.Tensor<
f32[128]
EXLA.Backend<host:0, 0.1357844422.1979580433.82183>
[0.0, NaN, NaN, NaN, NaN, NaN, NaN, NaN, 0.005036031361669302, NaN, NaN, NaN, NaN, NaN, NaN, NaN, NaN, NaN, NaN, NaN, NaN, NaN, NaN, NaN, NaN, NaN, NaN, NaN, NaN, NaN, NaN, NaN, NaN, NaN, NaN, NaN, NaN, NaN, NaN, NaN, NaN, NaN, NaN, NaN, NaN, NaN, ...]
>,
"kernel" => #Nx.Tensor<
f32[3][3][64][128]
EXLA.Backend<host:0, 0.1357844422.1979580433.82184>
[
[
[
[NaN, NaN, NaN, NaN, NaN, NaN, NaN, NaN, NaN, NaN, NaN, NaN, NaN, NaN, NaN, NaN, NaN, NaN, NaN, NaN, NaN, NaN, NaN, NaN, NaN, NaN, NaN, NaN, NaN, NaN, NaN, NaN, NaN, NaN, NaN, NaN, NaN, NaN, NaN, NaN, NaN, NaN, NaN, NaN, NaN, ...],
...
],
...
],
...
]
>
},
"dense_0" => %{
"bias" => #Nx.Tensor<
f32[128]
EXLA.Backend<host:0, 0.1357844422.1979580433.82185>
[NaN, -0.005992305930703878, -0.006005365401506424, -0.004664595704525709, NaN, NaN, NaN, -5.619042203761637e-4, 0.0, NaN, -0.005999671295285225, -6.131592726887902e-6, NaN, 0.0, NaN, 0.0, 0.0, NaN, NaN, -0.006002828478813171, -0.00600335793569684, 0.0, NaN, NaN, NaN, -0.006002923008054495, -0.006005282513797283, -0.00600528996437788, -0.0060048955492675304, -0.006004981696605682, NaN, -0.006004655733704567, -0.006005233619362116, NaN, -0.006004724185913801, -0.006005335133522749, -0.006005051080137491, -0.006004408933222294, NaN, -0.006005355156958103, 0.0, -0.006005344912409782, 0.0, NaN, -0.005991040728986263, ...]
>,
"kernel" => #Nx.Tensor<
f32[18432][128]
EXLA.Backend<host:0, 0.1357844422.1979580433.82186>
[
[NaN, NaN, NaN, NaN, NaN, NaN, NaN, NaN, NaN, NaN, NaN, NaN, NaN, NaN, NaN, NaN, NaN, NaN, NaN, NaN, NaN, NaN, NaN, NaN, NaN, NaN, NaN, NaN, NaN, NaN, NaN, NaN, NaN, NaN, NaN, NaN, NaN, NaN, NaN, NaN, NaN, NaN, NaN, NaN, ...],
...
]
>
},
"dense_1" => %{
"bias" => #Nx.Tensor<
f32[1]
EXLA.Backend<host:0, 0.1357844422.1979580433.82187>
[NaN]
>,
"kernel" => #Nx.Tensor<
f32[128][1]
EXLA.Backend<host:0, 0.1357844422.1979580433.82188>
[
[NaN],
[NaN],
[NaN],
[NaN],
[NaN],
[NaN],
[NaN],
[NaN],
[NaN],
[NaN],
[NaN],
[NaN],
[NaN],
[NaN],
[NaN],
[NaN],
[NaN],
[NaN],
[NaN],
[NaN],
[NaN],
[NaN],
[NaN],
[NaN],
[NaN],
[NaN],
[NaN],
[NaN],
[NaN],
[NaN],
[NaN],
[NaN],
[NaN],
[NaN],
[NaN],
[NaN],
[NaN],
[NaN],
[NaN],
[NaN],
[NaN],
[NaN],
[NaN],
...
]
>
}
}
The accuracy of the mlp_model was Batch: 6, accuracy: 0.5078125
and the accuracy of this cnn_model is Batch: 6, accuracy: 0.4944196
which was slightly worse instead of the expected “significantly better.”
I reviewed all the code to make sure I hadn’t missed anything, but I couldn’t find anything that didn’t match.
I’m guessing the NaN
s in the trained model state are a problem, but I’m not sure how to fix that.
Popular Prag Prog topics
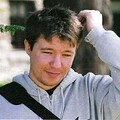
In Chapter 3, the source for index introduces Config on page 31, followed by more code including tests; Config isn’t introduced until pag...
New
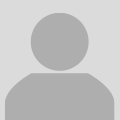
Hello Brian,
I have some problems with running the code in your book. I like the style of the book very much and I have learnt a lot as...
New
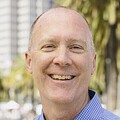
I thought that there might be interest in using the book with Rails 6.1 and Ruby 2.7.2. I’ll note what I needed to do differently here.
...
New
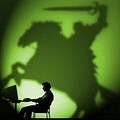
The generated iex result below should list products instead of product for the metadata. (page 67)
iex> product = %Product{}
%Pento....
New
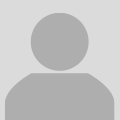
This isn’t directly about the book contents so maybe not the right forum…but in some of the code apps (e.g. turbo/06) it sends a TURBO_ST...
New
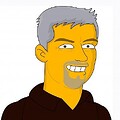
@noelrappin
Running the webpack dev server, I receive the following warning:
ERROR in tsconfig.json
TS18003: No inputs were found in c...
New
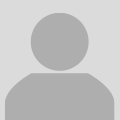
In general, the book isn’t yet updated for Phoenix version 1.6. On page 18 of the book, the authors indicate that an auto generated of ro...
New
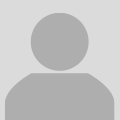
Skimming ahead, much of the following is explained in Chapter 3, but new readers (like me!) will hit a roadblock in Chapter 2 with their ...
New
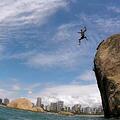
Book: Programming Phoenix LiveView, page 142 (157/378), file lib/pento_web/live/product_live/form_component.ex, in the function below:
d...
New
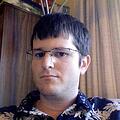
I’ve got to the end of Ch. 11, and the app runs, with all tabs displaying what they should – at first. After switching around between St...
New
Other popular topics
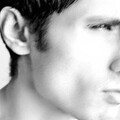
A thread that every forum needs!
Simply post a link to a track on YouTube (or SoundCloud or Vimeo amongst others!) on a separate line an...
New
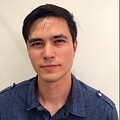
I’ve been really enjoying obsidian.md:
It is very snappy (even though it is based on Electron). I love that it is all local by defaul...
New
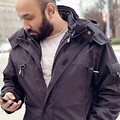
Crystal recently reached version 1. I had been following it for awhile but never got to really learn it. Most languages I picked up out o...
New
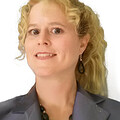
Think Again 50% Off Sale »
The theme of this sale is new perspectives on familiar topics.
Enter coupon code ThinkAgain2021 at checkout t...
New
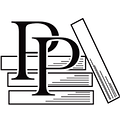
A Hero’s Journey
with Chris Pine
@chrispine
Chris Pine, author of Learn to Program, Third Edition, discusses his journey to beco...
New
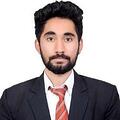
API 4
Path:
/user/following/
Method:
GET
Description:
Returns the list of all names of people whom the user follows
Response
[
{ ...
New
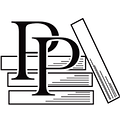
Author Spotlight
Dmitry Zinoviev
@aqsaqal
Today we’re putting our spotlight on Dmitry Zinoviev, author of Data Science Essentials in ...
New
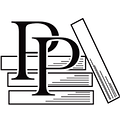
Author Spotlight
Jamis Buck
@jamis
This month, we have the pleasure of spotlighting author Jamis Buck, who has written Mazes for Prog...
New
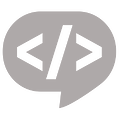
The overengineered Solution to my Pigeon Problem.
TL;DR: I built a wifi-equipped water gun to shoot the pigeons on my balcony, controlle...
New
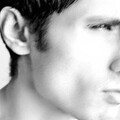
Chris Seaton, the creator of TruffleRuby has died. It appears from suicide :cry:
He left this note on Twitter on the weekend:
And one...
New
Latest in PragProg
Latest (all)
Categories:
Popular Portals
- /elixir
- /rust
- /wasm
- /ruby
- /erlang
- /phoenix
- /keyboards
- /js
- /rails
- /python
- /security
- /go
- /swift
- /vim
- /clojure
- /haskell
- /java
- /emacs
- /svelte
- /onivim
- /typescript
- /crystal
- /c-plus-plus
- /tailwind
- /kotlin
- /gleam
- /react
- /flutter
- /elm
- /ocaml
- /vscode
- /opensuse
- /centos
- /ash
- /php
- /deepseek
- /zig
- /scala
- /html
- /debian
- /nixos
- /lisp
- /agda
- /textmate
- /sublime-text
- /react-native
- /kubuntu
- /arch-linux
- /revery
- /ubuntu
- /manjaro
- /spring
- /django
- /diversity
- /nodejs
- /lua
- /slackware
- /julia
- /c
- /neovim